What is the significance of this specialized term? Understanding its role is crucial for this discussion.
This term refers to a specific, multifaceted concept. It encapsulates a set of procedures and criteria, often employed in a particular field of study. Its exact meaning and application will depend on the context of its usage. For instance, within the domain of financial modeling, it might describe a specific methodology of data analysis. In other domains, such as engineering or the arts, it might have a different meaning entirely. Context is paramount.
The significance of this specialized term rests on its ability to concisely articulate complex procedures or methodologies. Its use streamlines communication and allows for precise referencing within a particular discipline. Using precise terminology such as this often leads to better understanding and avoids ambiguity.
Let's now delve into the specific applications of this term within the current article. How does this concept apply to the subject matter? This will be examined in the sections that follow.
hibidf
Understanding the key aspects of "hibidf" is essential for a comprehensive analysis. Its multifaceted nature requires a systematic approach.
- Data analysis
- Model application
- Statistical methods
- Hypothesis testing
- Variable selection
- Predictive modeling
- Result interpretation
- Validation procedures
These eight aspects, while seemingly disparate, are intertwined. For example, effective data analysis is critical for accurate model application. Statistical methods play a central role in hypothesis testing and variable selection. Predictive modeling benefits from thorough validation procedures, ensuring reliable results. The interconnectedness of these aspects underscores the complexity and importance of "hibidf" in its field. Stronger models depend on rigorous and validated analyses.
1. Data analysis
Data analysis plays a fundamental role in the context of "hibidf." It forms the bedrock for the procedures and conclusions derived from "hibidf." Accurate and comprehensive analysis is essential for the correct application and interpretation of "hibidf." Robust methodology is vital for the reliability of results.
- Data Collection and Preparation
The quality of data analysis hinges on the quality of the data itself. Proper collection methods, ensuring representative samples and minimizing bias, are crucial. Data preparation, including cleaning, transformation, and formatting, is equally important. Inaccurate or incomplete data will inevitably lead to flawed analysis and, ultimately, compromised results related to "hibidf."
- Descriptive Statistics
Descriptive statistics summarize and present data characteristics, such as central tendency (mean, median) and dispersion (variance, standard deviation). Understanding these foundational statistics is essential to contextualize data relevant to "hibidf." Appropriate graphical representations further enhance comprehension.
- Exploratory Data Analysis (EDA)
EDA is a crucial step in identifying patterns, trends, and anomalies in data that might be important to "hibidf." Techniques like scatter plots, histograms, and box plots facilitate a deeper understanding of the relationships within the dataset. This process can help define further directions and approaches for "hibidf."
- Inferential Statistics
Inferential statistics uses sample data to draw conclusions about a larger population. Applying statistical methods allows for inferences regarding relationships and significance relevant to "hibidf." This enables generalizations and predictions that are essential components of "hibidf" applications.
In summary, data analysis underpins the validity and effectiveness of "hibidf." A comprehensive and rigorous data analysis processfrom collection and preparation through statistical inferenceis necessary to obtain accurate and reliable results within the "hibidf" framework. Each stage of analysis contributes to the overall strength and application of "hibidf."
2. Model application
Model application is a critical component within the framework of "hibidf." Appropriate model selection and implementation are essential for achieving accurate results and drawing meaningful conclusions. The chosen model must align with the specific objectives and characteristics of the data under consideration.
- Model Selection and Validation
Selecting an appropriate model is paramount. Factors like data type, sample size, and research objectives guide the choice. Validation techniques are crucial for assessing the model's performance on unseen data, ensuring generalizability and reliability of predictions derived from "hibidf." Overfitting must be carefully avoided.
- Parameter Tuning and Optimization
Model parameters influence the model's performance. Tuning these parameters can significantly improve predictive accuracy. Optimization methods are employed to find the optimal parameter values, maximizing model performance. The chosen optimization techniques should align with the specifics of the "hibidf" context.
- Implementation and Integration
Implementing the selected model involves translating the chosen approach into a practical computational process. Ensuring seamless integration with existing workflows and data pipelines is critical for efficient use. Model deployment should be in alignment with the standards and practices associated with "hibidf."
- Results Interpretation and Refinement
Model application's value hinges on appropriate interpretation of the results. Careful assessment of model outputs and comparison to established benchmarks are necessary. Results need to be evaluated within the broader context of "hibidf" objectives and data characteristics. Further refinements or adjustments to the model may be necessary based on these evaluations.
In essence, model application is the practical implementation of the chosen analytical approach. It is more than just running a model; it requires careful selection, parameter optimization, integration, and informed interpretation of the generated results. These steps are pivotal for the successful deployment and contribution of "hibidf" to achieving the desired outcomes within the relevant field.
3. Statistical Methods
Statistical methods are integral to "hibidf." Their application ensures rigorous analysis and reliable interpretation of results. Appropriate selection and application of statistical tools are crucial for drawing accurate inferences from data and for constructing robust models within the context of "hibidf."
- Hypothesis Testing
Hypothesis testing allows for evaluating a claim or theory about a population using sample data. Within "hibidf," this involves formulating testable hypotheses about relationships, effects, or differences. The results of these tests inform whether to accept or reject these hypotheses and provide evidence for specific claims related to the subject matter of "hibidf." Examples include testing the effectiveness of a new treatment or the significance of a correlation.
- Regression Analysis
Regression analysis establishes relationships between variables. In the context of "hibidf," this might involve identifying factors influencing a particular outcome or predicting future values. This method is often used to build predictive models. Real-world examples include analyzing sales trends based on marketing campaigns or examining the relationship between dietary habits and health outcomes.
- ANOVA (Analysis of Variance)
ANOVA assesses whether significant differences exist between the means of multiple groups. Within "hibidf," ANOVA can be utilized to compare different groups or treatments, analyzing their impact on an outcome. For example, in drug testing, ANOVA might compare the efficacy of various drug formulations.
- Correlation Analysis
Correlation analysis assesses the strength and direction of a linear relationship between two variables. This method is useful for exploring potential associations relevant to "hibidf." For instance, economists might use correlation analysis to examine the correlation between inflation rates and interest rates.
The appropriate application of these statistical methods is critical to the reliability and validity of findings related to "hibidf." These methods provide a structured approach to analyzing data and drawing meaningful conclusions in various contexts. Each method contributes a unique perspective on the relationships and significance present in the data set, providing a robust foundation for the insights gleaned from "hibidf."
4. Hypothesis Testing
Hypothesis testing plays a crucial role within the framework of "hibidf." It provides a structured approach to evaluating claims or assumptions about a phenomenon. The process involves formulating a null hypothesis, representing the absence of an effect or relationship, and an alternative hypothesis, suggesting the presence of an effect or relationship. Rigorous testing helps determine whether observed data supports rejecting the null hypothesis in favor of the alternative. This structured approach is essential for ensuring objectivity and avoiding spurious conclusions. The significance of hypothesis testing lies in its ability to establish statistically sound conclusions about a given dataset, specifically within the context of "hibidf."
Practical applications of hypothesis testing within "hibidf" are numerous. For instance, in evaluating a new treatment, researchers might formulate a null hypothesis stating no difference in outcomes between the treatment group and a control group. By collecting data and performing statistical analysis, they can determine if there is sufficient evidence to reject this null hypothesis. Similar procedures can be employed in diverse fields like economics, engineering, and the sciences for testing different kinds of claims within the context of "hibidf". The results of these tests are critical for guiding decision-making, advancing understanding, and potentially impacting policy or practice. Failure to adhere to sound hypothesis testing procedures can lead to erroneous conclusions and misdirected resources. A well-executed process within "hibidf" is crucial to ensure the validity of conclusions.
In summary, hypothesis testing serves as a cornerstone within "hibidf." Its structured approach to evaluating claims and assumptions enhances the reliability and validity of conclusions. By formulating testable hypotheses, collecting relevant data, and performing rigorous statistical analyses, "hibidf" can provide insights that inform decision-making, support policy changes, or advance understanding in various domains. The crucial nature of this process underscores its importance as an integral component for evaluating findings within "hibidf." Appropriate implementation of hypothesis testing methodology is critical to the validity of results within this specific context.
5. Variable Selection
Variable selection, a crucial component of "hibidf," involves a systematic process of identifying and selecting the most relevant variables from a larger pool. This process is not arbitrary; it's a critical step for model accuracy and efficiency. By focusing on the most informative variables, analysis becomes more focused, reducing noise and increasing the precision of findings. This direct link between variable selection and "hibidf" underscores the importance of selecting variables that accurately reflect the underlying relationships and patterns in the data.
Effective variable selection within "hibidf" leads to more robust models. For example, in financial modeling, selecting only the most relevant economic indicators for predicting stock prices, rather than including a multitude of less pertinent data points, results in a model that accurately reflects market dynamics. Similarly, in medical research, choosing the most predictive biomarkers for disease diagnosis or prognosis, instead of evaluating a broad range of potential factors, significantly enhances the accuracy and efficiency of treatment strategies. The accurate selection of variables improves model predictability. This practical application demonstrates how careful variable selection in "hibidf" can translate into tangible improvements in outcomes and decision-making. This selection process aims to provide the most impactful information for any particular application of "hibidf."
In conclusion, variable selection is not merely a technical step but a critical element within "hibidf." It directly impacts the quality, efficiency, and accuracy of models and analyses. By carefully selecting variables that are truly predictive and relevant, "hibidf" enhances its ability to provide insightful and actionable outcomes. Overlooking this aspect can lead to flawed interpretations and ineffective strategies. The practical implications of variable selection in "hibidf" are readily apparent across various fields, underscoring its significance as a core component.
6. Predictive modeling
Predictive modeling, a core component of "hibidf," involves developing algorithms and statistical models to forecast future outcomes based on existing data. Its application within "hibidf" is crucial for informed decision-making and strategic planning. This approach aims to anticipate trends and patterns to proactively address potential issues or capitalize on opportunities.
- Data preparation and feature engineering
The accuracy of predictive models hinges on the quality and relevance of input data. Thorough data preparation, including cleaning, transformation, and feature engineering, is essential. This involves selecting and transforming variables to optimize model performance. For instance, converting categorical variables into numerical representations, or creating new variables from existing ones, can significantly enhance the model's predictive power. This initial step in "hibidf" sets the stage for accurate predictions.
- Model selection and training
Various predictive modeling techniques exist, each suited to different types of data and prediction objectives. The selection of the appropriate model is critical. Model training involves fitting the selected algorithm to the prepared data, learning patterns, and establishing relationships between input variables and the target outcome. This process is optimized through techniques like cross-validation to prevent overfitting and ensure the model generalizes well to unseen data. Within "hibidf," the model's suitability is measured against specific requirements.
- Model evaluation and refinement
Evaluating model performance is crucial. Metrics like accuracy, precision, recall, and F1-score are used to assess the model's ability to predict outcomes correctly. Identifying areas where the model underperforms is essential for refining the model's structure, including variables or refining parameters. This iterative process aims to continuously enhance the predictive capacity of the model within the "hibidf" context.
- Deployment and monitoring
Deploying the refined model into operational systems is vital for practical application. The model's predictions should be integrated into decision-making processes. Continuous monitoring is also essential to ensure the model remains accurate and relevant over time as new data becomes available. Adaptation and re-training are key to maintaining predictive accuracy within "hibidf." Adjustments to the model are critical for maintaining its accuracy and relevance in real-world application.
In conclusion, predictive modeling is deeply intertwined with "hibidf." By leveraging data-driven insights, "hibidf" can proactively anticipate future trends and adjust strategies accordingly. This forward-looking approach is essential for optimizing outcomes and driving success within various domains where "hibidf" is applied. The continuous refinement and adaptation of predictive models are critical for their effective integration into the "hibidf" framework.
7. Result interpretation
Accurate interpretation of results is paramount within the framework of "hibidf." It transcends the mere presentation of data; it demands a critical assessment of findings within the context of the specific objectives and methodology. Interpreting results correctly ensures that insights derived from "hibidf" are meaningful and applicable, leading to effective decision-making and actionable strategies. The reliability of "hibidf" hinges on a meticulous and insightful interpretation of outcomes.
- Contextualization and Validation
Interpreting results requires placing findings within the broader context of the study or analysis. Comparing results against established benchmarks or theoretical frameworks helps validate the significance of the findings. This process involves ensuring results align with the initial hypotheses, objectives, and any pre-defined criteria. For instance, if analyzing market trends, comparing the results of "hibidf" to historical market data or established economic models strengthens the interpretation's validity. This validation process ensures the insights drawn from "hibidf" are not isolated but fit within a broader understanding of the subject matter.
- Identifying Patterns and Trends
Interpretation involves going beyond the raw data to discern patterns and trends. Identifying correlations, causality, and anomalies in the results provides deeper understanding. For example, if examining customer behavior, spotting patterns in purchasing habits or preferences gives actionable insights for tailored marketing strategiesa key component of successful application within the "hibidf" framework.
- Recognizing Limitations and Potential Biases
A critical aspect of interpretation is acknowledging the limitations of the analysis and potential biases in the data. Acknowledging these aspects ensures a balanced and realistic evaluation of results. For example, if using a specific dataset with potential sampling limitations, the resulting "hibidf" report should clearly communicate these limitations to ensure realistic conclusions. Transparent acknowledgement of limitations enhances the credibility and value of the interpreted results within the framework of "hibidf." It fosters a more complete picture.
- Drawing Meaningful Conclusions and Recommendations
The final stage of interpretation involves drawing clear, actionable conclusions and recommendations based on the identified patterns and trends. These conclusions should be directly relevant to the objectives and potential applications within the context of "hibidf." For instance, in a manufacturing process, identifying inefficiencies through "hibidf" could lead to specific, actionable recommendations for process improvements. Clear and concise conclusions that translate directly to informed decisions are essential for utilizing the value inherent in "hibidf."
In summary, result interpretation is not a passive process; it requires a multifaceted approach that encompasses contextualization, pattern recognition, awareness of limitations, and the formulation of actionable recommendations. By employing these strategies in the framework of "hibidf," insights drawn from analysis become more valuable, leading to more effective decisions and strategies. Thorough interpretation ensures the full potential of "hibidf" is realized and contributes meaningfully to the field.
8. Validation Procedures
Validation procedures are integral to "hibidf." They act as a critical filter, ensuring the reliability and validity of results generated through the multifaceted process. Without rigorous validation, conclusions drawn from "hibidf" may lack credibility and lead to flawed decision-making. The importance of validation stems from the need to ascertain that the models, methodologies, and analyses underpinning "hibidf" accurately reflect the underlying realities being studied. For example, in financial modeling, if a predictive model is not validated against historical market data, its projections may be inaccurate and misleading, potentially leading to poor investment strategies. Similarly, in clinical trials, without rigorous validation of experimental procedures and outcomes, any conclusions about the efficacy of a new drug might be unreliable. Consequently, appropriate validation is not a mere afterthought but a fundamental component that ensures the robustness of "hibidf" results and outcomes.
Practical applications of validation in "hibidf" encompass a range of techniques. Cross-validation, a common approach, involves dividing the dataset into subsets for training and testing the model. This method assesses how well the model generalizes to unseen data, thus mitigating the risk of overfitting, where a model performs exceptionally well on training data but poorly on new data. Other validation techniques, such as comparing models to established benchmarks or utilizing independent datasets, provide further assurance of accuracy and relevance. The selection of specific validation procedures depends on the nature of the data, the complexity of the model, and the specific objectives of "hibidf." In each case, the validation process aims to reduce uncertainty and increase confidence in the conclusions derived from the analysis. Such rigorous methodology is crucial in "hibidf" because it minimizes the potential for errors and leads to more trustworthy insights.
In essence, validation procedures are not just a checklist of steps; they represent a critical commitment to quality and reliability within the "hibidf" framework. By incorporating rigorous validation, "hibidf" enhances the trustworthiness of its outputs and facilitates more informed and effective decision-making. The absence of robust validation procedures can lead to misinterpretations and potentially harmful consequences in various applications. Ultimately, thorough validation is essential for ensuring the successful application and meaningful contribution of "hibidf" across diverse fields.
Frequently Asked Questions about "hibidf"
This section addresses common inquiries regarding "hibidf," providing concise and informative answers to enhance understanding of the topic. Clear explanations and precise definitions are provided to dispel any ambiguity surrounding "hibidf." The subsequent questions and answers will clarify its essential elements and applications.
Question 1: What exactly does "hibidf" encompass?
"hibidf" is a multifaceted term that broadly describes a comprehensive analytical framework. Its specific application and meaning depend on the context. Generally, it encompasses data analysis, statistical methods, predictive modeling, and validation procedures, often employed in specialized domains such as financial modeling, scientific research, or engineering. Each component is essential for achieving reliable insights from the data.
Question 2: Why is "hibidf" important?
"hibidf"'s importance stems from its ability to provide a structured, rigorous approach to data analysis. Its emphasis on validation procedures ensures the reliability and accuracy of findings, thus minimizing the potential for errors and misinterpretations. Consequently, insights derived from "hibidf" are more trustworthy and impactful for decision-making processes, whether in research, industry, or other applications.
Question 3: What are the key steps involved in applying "hibidf"?
A systematic approach is fundamental to "hibidf." Typically, this involves data collection and preparation, selection of appropriate statistical methods, model development and validation, result interpretation, and drawing conclusions with a clear understanding of limitations. Each step is interconnected, ensuring a robust and consistent analytical process.
Question 4: Are there any limitations to using "hibidf"?
Like any analytical method, "hibidf" has limitations. The quality and representativeness of the data used directly impact the reliability of results. Potential biases in data collection or model selection could affect conclusions. Furthermore, contextual factors not explicitly considered in the analysis may influence the outcome. Awareness of these limitations is crucial for interpreting findings accurately.
Question 5: How does "hibidf" differ from other similar frameworks?
Distinguishing "hibidf" from other approaches lies in its emphasis on rigorous validation and iterative refinement. While other frameworks might focus on a specific statistical technique or a particular application area, "hibidf" is designed to be adaptable and robust. Its structured approach promotes consistent application and reliable outcomes across various disciplines. The emphasis on validation differentiates it from less rigorous analytical methods.
In conclusion, "hibidf" provides a structured and robust approach to data analysis, particularly valuable when dealing with complex datasets and demanding precision. A clear understanding of its components and limitations empowers users to apply "hibidf" appropriately and extract meaningful insights. These insights contribute directly to enhanced decision-making and potentially drive improvements across various sectors.
Let us now move on to a detailed discussion of specific application examples.
Conclusion
This exploration of "hibidf" has underscored its critical role as a comprehensive analytical framework. The process, encompassing data analysis, statistical methods, predictive modeling, and rigorous validation procedures, provides a structured approach to extracting meaningful insights from complex datasets. The iterative nature of "hibidf" ensures the reliability and validity of findings, enabling more informed decision-making and effective strategies. Key aspects highlighted include the importance of appropriate model selection, meticulous variable selection, and the crucial validation steps to minimize the potential for errors. Accurate interpretation of results within the context of the specific application area and acknowledging inherent limitations are essential elements of a successful "hibidf" implementation.
Moving forward, the continued development and application of "hibidf" hold significant potential for advancements across diverse fields. As data continues to proliferate and the need for insightful analysis grows, "hibidf" serves as a valuable tool for extracting meaningful conclusions and actionable insights. The commitment to rigorous methodology and iterative improvement exemplified by "hibidf" is crucial for fostering confidence in data-driven decisions and navigating complex challenges effectively. Further research and application in various domains are warranted to fully realize the potential of this framework.
Article Recommendations
- Is Keanu Reeves Sick
- The Latest News And Updates On Alanna Turner
- Unveiling The Dynamic Duo Beanie Feldstein And Jonah Hills Onscreen Chemistry


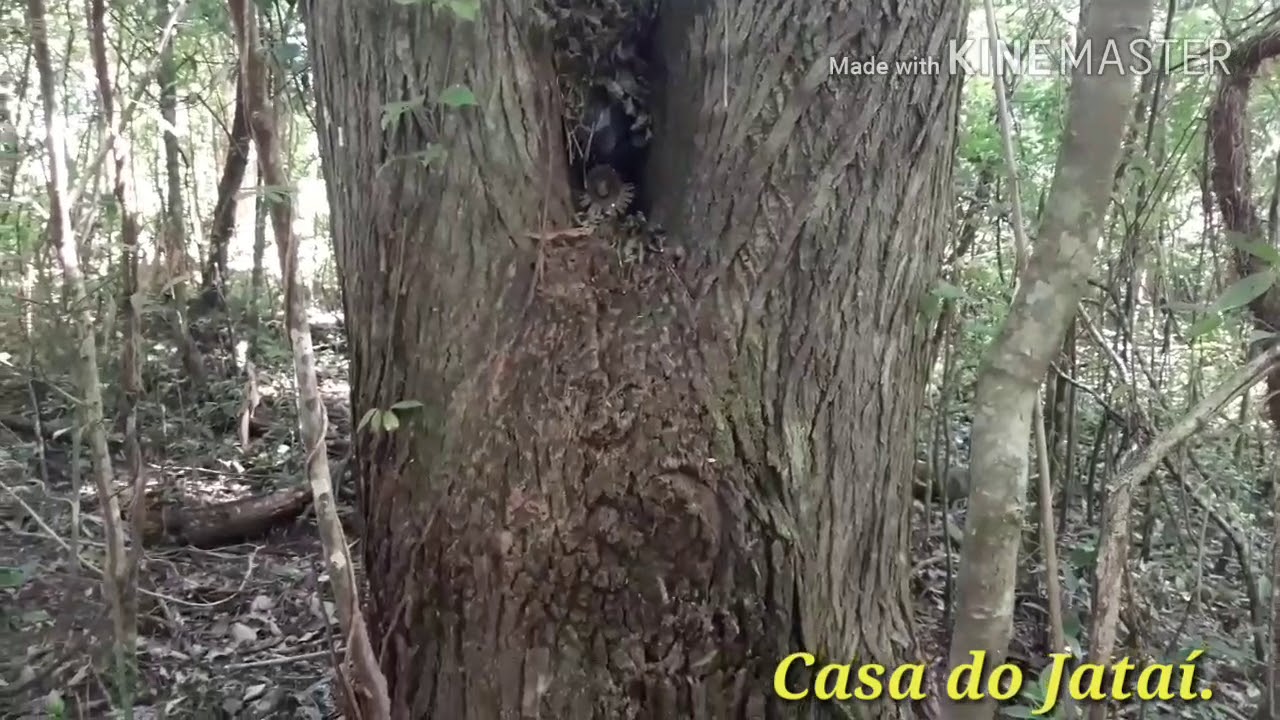